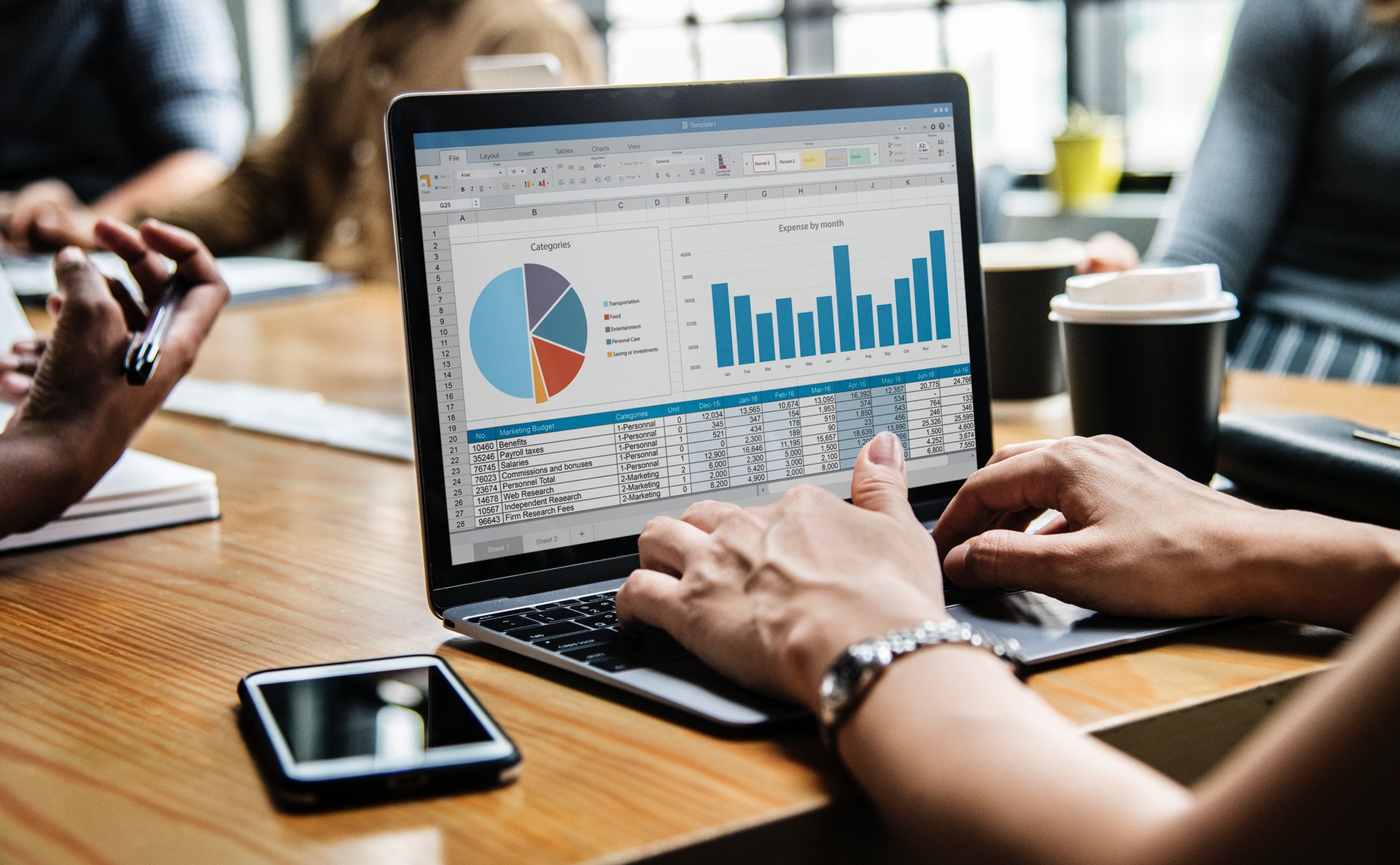
“Big Data” was one of the hottest terms in business technology a few years back. One of the major drivers behind “big data” was the increase of internet-connected devices that allowed businesses to grow and diversify complex datasets around device usage.
Since this time, there has been an accelerated boom in the number of internet-connected devices worldwide. In fact, it’s gone from approximately 15 billion in 2015 to more than 23 billion in 2018!
And it’s predicted that number will reach over 75 billion by 2025.
This growth has major value implications for businesses, and many businesses are already using their vast data repositories as means of setting valuation and leveraging further financial gain through data-sharing partnerships and reselling analytics to suppliers and customers.
However, managing this “Internet of Things” (IoT) is quite challenging, as these types of datasets are often too complex, large, and fluid for a human to effectively interpret.
The solution to this problem is one of the most significant advances in recent business technology: machine learning, which allows businesses to understand, interpret, and act on these vast datasets.
Create Customer-Centric Product Roadmaps
When it comes to implementing machine learning in your business, the key to success is to create a product roadmap that meets the needs of the customer.
For example, device data can be collected and analyzed by machine learning algorithms to pinpoint the most- and least-used features of a software application or mobile app.
This insight is especially effective when it comes to releasing new updates and features, as it allows you to effectively crowd-source your ongoing development without expensive user research studies.
Another example would be to use machine learning algorithms to adapt to changing market conditions on the fly. As new trends and conditions are detected, machine learning can help you identify these trends and make recommendations for improvement—for example, recommending new configurations to the end user based on the success of others.
Use Machine Learning to Detect Anomalies Early
Nine times out of ten, keeping devices and equipment up and running revolves around telemetry data—things like log messages and measurements (connection speed, battery status, temperature, etc.).
The ability to detect issues and problems without manually monitoring telemetry data is hugely valuable for businesses, and allows you to be proactive in resolving issues before you hear about them from your customers.
Machine learning could help you detect whether devices on a particular software version or connection type tend to fail faster, allowing you to perform predictive maintenance to patch or upgrade systems before they fail.
Security is probably the most relevant way that machine learning can help businesses today detect intrusion or fraudulent activity.
For example, the temperature of a business’s sensors may all vary in a similar manner. So when one sensor is too static, it could indicate a security compromise or another anomaly. Or, machine learning could detect a device sending massive amounts of data significantly above the activity of similar devices.
Energize Data-Driven Decision Making
Techniques like neuro-linguistic programming (NLP), unstructured data, and digitizing and consuming paper documents are only useful if a real person can ultimately use these methods and make decisions based on the results. This is a key driver behind why machine learning is now focusing not just on the data analysis but also on how to make human interaction with data easier.
Natural language processing has become a larger part of solution design and now allows humans to ask relevant and compelling questions of machine learning algorithms. A user might ask which product line generated the highest profits in Q2 of 2018, and machine learning could generate a chart of profitability by product in Q2 2018.
Another exciting area developing in machine learning is through helping improve the ability to make data-driven decisions through the breakdown and consumption of unstructured data.
This would typically involve something like a Facebook feed or other highly varied text-based information source. Machine learning can take unstructured data like this and identify common elements and trends in the text. This is useful for monitoring things like user sentiment towards your products and services.
Finally, an obvious, but often-overlooked, way that machine learning can energize a data-driven culture is by helping your business digitize without disrupting normal business operations.
The primary mechanism for this is using optical character recognition (OCR) to turn scanned documents into digitally readable and searchable documents. This allows for digital archiving, which saves physical space and creates a reliable method for future retrieval.
Develop 21st Century Thinking
Every business needs to have a plan for how digital information can help drive decision making. Hardware is rapidly becoming cheaper and more readily available through cloud providers, and software is becoming more powerful and efficient.
Leveraging machine learning to analyze data even just 5-10 years ago would have been a major undertaking, but today implementing a machine learning solution takes relatively little upfront capital and can sometimes be implemented in as little as a few days.
The trend towards data-driven decision making started decades ago with the development of software like Microsoft Excel, and more recently through concepts like data warehousing.
As with most technologies, data analysis is evolving rapidly, and businesses that refuse to evolve and adopt a new mindset will eventually lose ground to more forward-thinking competitors who know how to use solutions like machine learning to their advantage.
So, how will your business adapt?